Human Pose, Shape and Action
3D Pose from Images
2D Pose from Images
Beyond Motion Capture
Action and Behavior
Body Perception
Body Applications
Pose and Motion Priors
Clothing Models (2011-2015)
Reflectance Filtering
Learning on Manifolds
Markerless Animal Motion Capture
Multi-Camera Capture
2D Pose from Optical Flow
Body Perception
Neural Prosthetics and Decoding
Part-based Body Models
Intrinsic Depth
Lie Bodies
Layers, Time and Segmentation
Understanding Action Recognition (JHMDB)
Intrinsic Video
Intrinsic Images
Action Recognition with Tracking
Neural Control of Grasping
Flowing Puppets
Faces
Deformable Structures
Model-based Anthropometry
Modeling 3D Human Breathing
Optical flow in the LGN
FlowCap
Smooth Loops from Unconstrained Video
PCA Flow
Efficient and Scalable Inference
Motion Blur in Layers
Facade Segmentation
Smooth Metric Learning
Robust PCA
3D Recognition
Object Detection
Bodies in Medicine
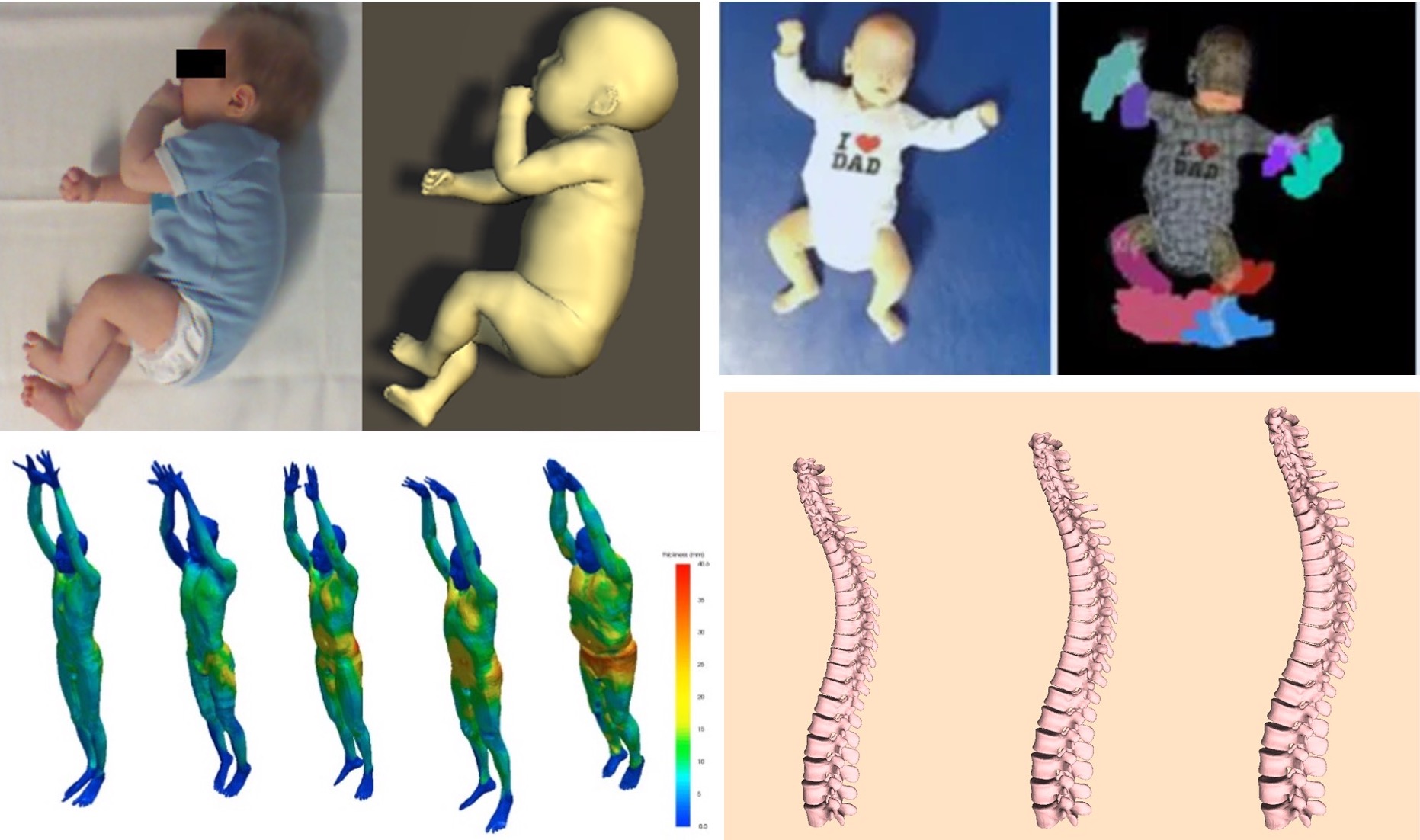
Body shape and movement are related to human health. Using our 3D models of body shape we analyze movement and shape to create non-invasive and deployable methods for analyzing human health.
For example, if Cerebral Palsy (CP) is detected early, there are effective therapies to minimize the impact in later life. CP can be diagnosed in infants based on their spontaneous, undirected movements. Unfortunately, this requires expert training that is not widely available. By automatically tracking infant movement, we can automate the early detection of CP. Methods for tracking adult 3D motion, however, do not work for infants due to their very different body shape.
Since we lack 3D scans of infants, we developed a novel method that learns an infant body shape model, called SMIL, directly from low quality, incomplete, RGB-D scan sequences and deployed this in hospitals where we scanned over 30 infants []. Using SMIL we track spontaneous infant movements in RGB-D sequences and train a method to automatically recognize pathological movements [
].
We also train methods to predict what is inside the body by observing the outside. Take, for example, the distribution of body fat. Visceral adipose tissue is correlated with disease while sub-cutaneous adipose tissue is relatively benign. We are developing methods to estimate this fat distribution purely from the surface shape of the body. To that end, we fit our 3D body models to full-body MRI scans [] to model both the external surface and the subcutaneous fat layer.
To make predictions of the body composition of a person from a surface scan, a main challenge lies in the variance of the body shape due to the pose. In collaboration with the University of Hawai'i Cancer Center (US) we show how SMPL allows us to factor out pose variations from surface scan data, improving the accuracy of the body composition estimation [].
Members
Publications