Human Pose, Shape and Action
3D Pose from Images
2D Pose from Images
Beyond Motion Capture
Action and Behavior
Body Perception
Body Applications
Pose and Motion Priors
Clothing Models (2011-2015)
Reflectance Filtering
Learning on Manifolds
Markerless Animal Motion Capture
Multi-Camera Capture
2D Pose from Optical Flow
Body Perception
Neural Prosthetics and Decoding
Part-based Body Models
Intrinsic Depth
Lie Bodies
Layers, Time and Segmentation
Understanding Action Recognition (JHMDB)
Intrinsic Video
Intrinsic Images
Action Recognition with Tracking
Neural Control of Grasping
Flowing Puppets
Faces
Deformable Structures
Model-based Anthropometry
Modeling 3D Human Breathing
Optical flow in the LGN
FlowCap
Smooth Loops from Unconstrained Video
PCA Flow
Efficient and Scalable Inference
Motion Blur in Layers
Facade Segmentation
Smooth Metric Learning
Robust PCA
3D Recognition
Object Detection
Pose and Motion Priors
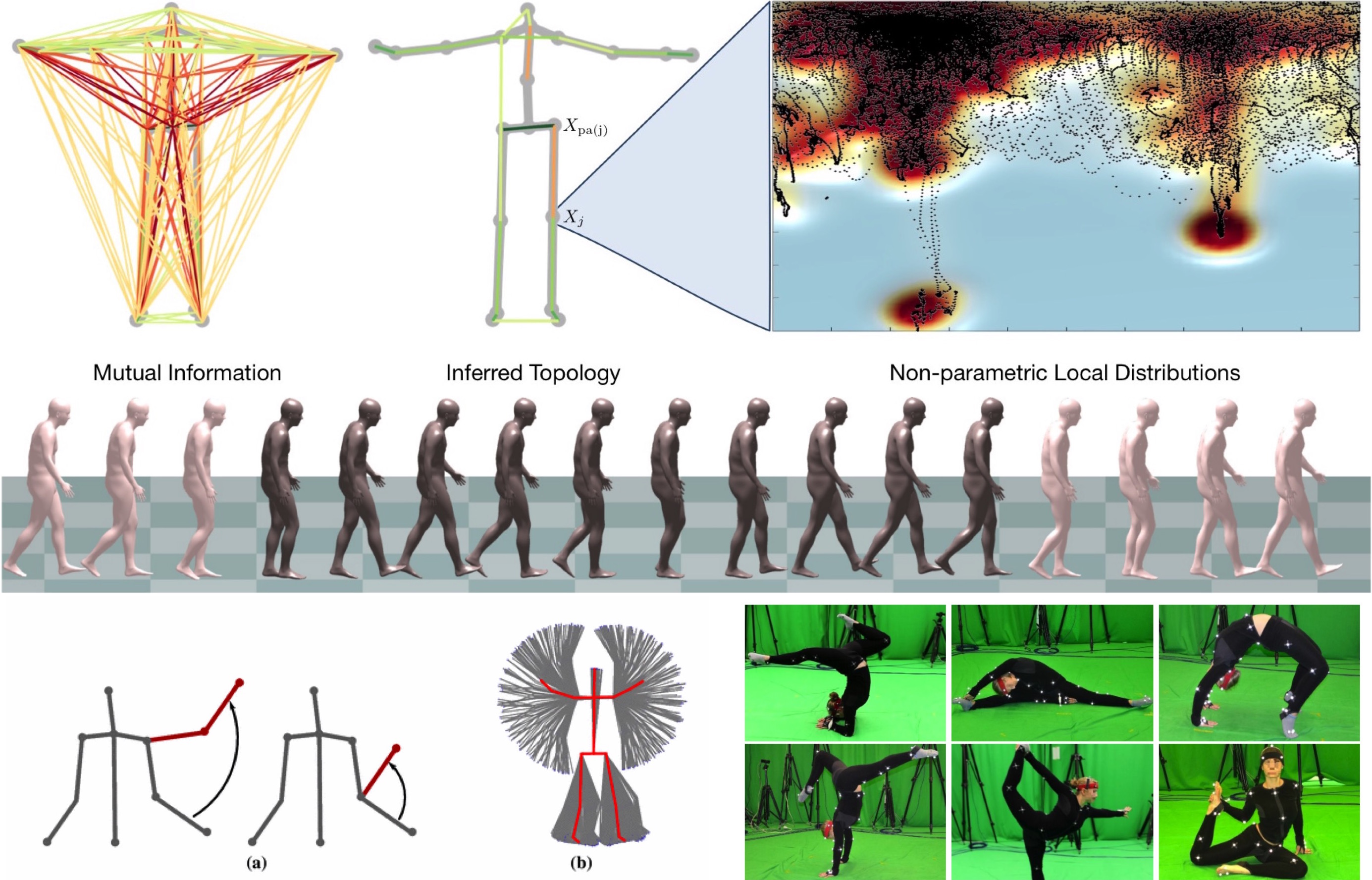
A prior over human pose is important for many human tracking and pose estimation problems.
We introduce a sparse Bayesian network model of human pose that is non-parametric with respect to the estimation of both its graph structure and its local distributions []. Using an efficient sampling scheme, we tractably compute exact log-likelihoods. The model is compositional, representing poses not present in the training set. It remains useful for real-time inference despite being non-parametric.
Action recognition and pose estimation are closely related topics; information from one task can be leveraged to assist the other, yet the two are often treated separately. In [] we develop a framework for coupled action recognition and pose estimation by formulating pose estimation as an optimization over a set of action-specific manifolds. The framework allows for integration of a 2D appearance-based action recognition system as a prior for 3D pose estimation and for refinement of the action labels using relational pose features based on the extracted 3D poses.
Modeling distributions over human poses requires a distance measure between human poses; this is often taken to be the Euclidean distance between joint angle vectors. In [] we present an algorithm for computing geodesics in the Riemannian space of joint positions, as well as a fast approximation that allows for large-scale analysis. Articulated tracking systems can be improved by replacing the standard distance with the geodesic distance in the space of joint positions. This measure significantly outperforms the traditional measure in classification, clustering and dimensionality reduction tasks.
To better model human pose we collected a new motion capture dataset of extreme poses [] that is available to the public.
Members
Publications