Human Pose, Shape and Action
3D Pose from Images
2D Pose from Images
Beyond Motion Capture
Action and Behavior
Body Perception
Body Applications
Pose and Motion Priors
Clothing Models (2011-2015)
Reflectance Filtering
Learning on Manifolds
Markerless Animal Motion Capture
Multi-Camera Capture
2D Pose from Optical Flow
Body Perception
Neural Prosthetics and Decoding
Part-based Body Models
Intrinsic Depth
Lie Bodies
Layers, Time and Segmentation
Understanding Action Recognition (JHMDB)
Intrinsic Video
Intrinsic Images
Action Recognition with Tracking
Neural Control of Grasping
Flowing Puppets
Faces
Deformable Structures
Model-based Anthropometry
Modeling 3D Human Breathing
Optical flow in the LGN
FlowCap
Smooth Loops from Unconstrained Video
PCA Flow
Efficient and Scalable Inference
Motion Blur in Layers
Facade Segmentation
Smooth Metric Learning
Robust PCA
3D Recognition
Object Detection
Generative Proxemics: A Prior for 3D Social Interaction from Images
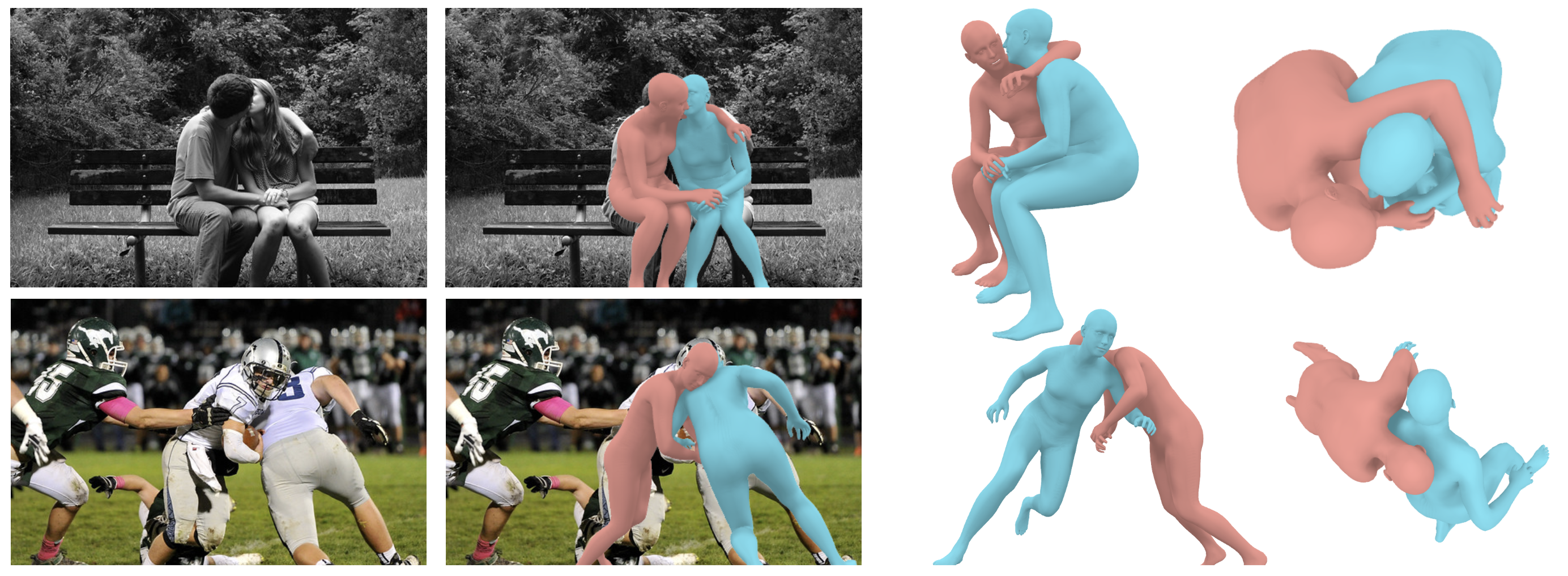
Social interaction is a fundamental aspect of human behavior and communication. The way individuals position themselves in relation to others, also known as proxemics, conveys social cues and affects the dynamics of social interaction. Reconstructing such interaction from images presents challenges because of mutual occlusion and the limited availability of large training datasets. To address this, we present a novel approach that learns a prior over the 3D proxemics two people in close social interaction and demonstrate its use for single-view 3D reconstruction. We start by creating 3D training data of interacting people using image datasets with contact annotations. We then model the proxemics using a novel denoising diffusion model called BUDDI that learns the joint distribution over the poses of two people in close social interaction. Sampling from our generative proxemics model produces realistic 3D human interactions, which we validate through a perceptual study. We use BUDDI in reconstructing two people in close proximity from a single image without any contact annotation via an optimization approach that uses the diffusion model as a prior. Our approach recovers accurate and plausible 3D social interactions from noisy initial estimates, outperforming state-of-the-art methods.
Members
Publications