Human Pose, Shape and Action
3D Pose from Images
2D Pose from Images
Beyond Motion Capture
Action and Behavior
Body Perception
Body Applications
Pose and Motion Priors
Clothing Models (2011-2015)
Reflectance Filtering
Learning on Manifolds
Markerless Animal Motion Capture
Multi-Camera Capture
2D Pose from Optical Flow
Body Perception
Neural Prosthetics and Decoding
Part-based Body Models
Intrinsic Depth
Lie Bodies
Layers, Time and Segmentation
Understanding Action Recognition (JHMDB)
Intrinsic Video
Intrinsic Images
Action Recognition with Tracking
Neural Control of Grasping
Flowing Puppets
Faces
Deformable Structures
Model-based Anthropometry
Modeling 3D Human Breathing
Optical flow in the LGN
FlowCap
Smooth Loops from Unconstrained Video
PCA Flow
Efficient and Scalable Inference
Motion Blur in Layers
Facade Segmentation
Smooth Metric Learning
Robust PCA
3D Recognition
Object Detection
Hands-Object Interaction
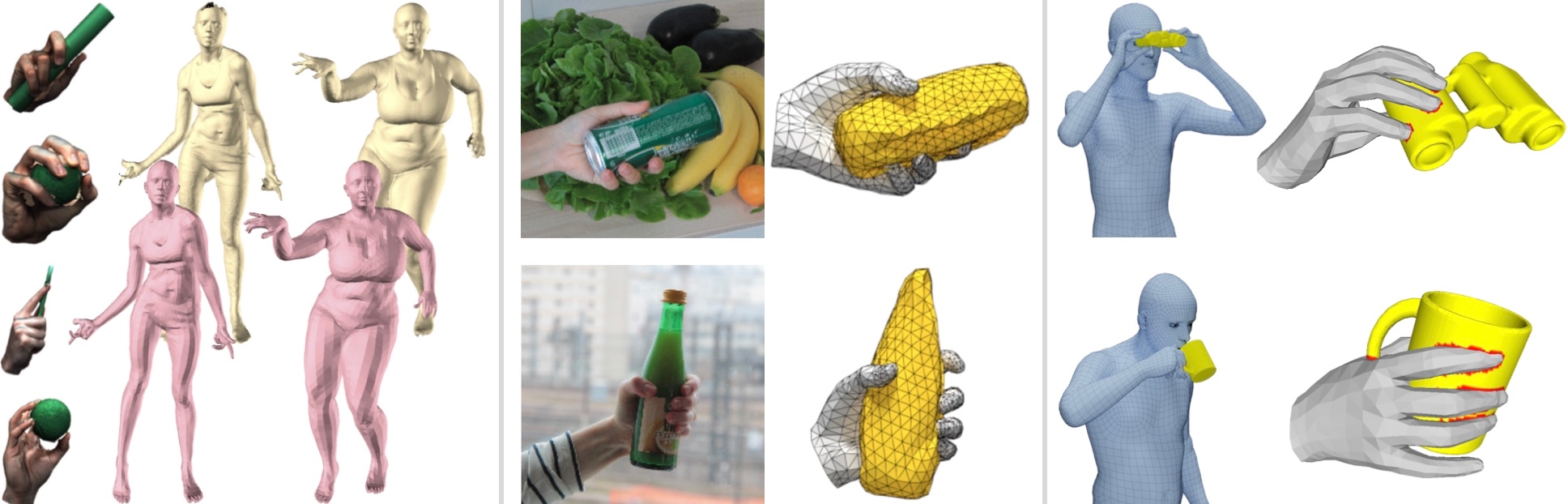
Hands allow humans to interact with, and use, physical objects, but capturing hand motion is a challenging computer-vision task. To tackle this, we learn a statistical model of the human hand [], called MANO, that is trained using many 3D scans of human hands and represents the 3D shape variation across a human population. We combine MANO with the SMPL body model and FLAME face model to obtain the expressive SMPL-X model, which allows us to reconstruct realistic bodies and hands using our 4D scanner, mocap data, or monocular video.
MANO can be fit to noisy input data to reconstruct hands and/or objects [] from a monocular RGB-D or multiview RGB sequence. Interacting motion also helps to recover the unknown kinematic skeleton of objects [
].
To directly regress hands and objects, we developed ObMan [], a deep-learning model that integrates MANO as a network layer, to estimate the 3D hand and object meshes from an RGB image of grasping. For training data, we use MANO and ShapeNet objects to generate synthetic images of hand-object grasps. ObMan's joint hand-object reconstruction allows the network to encourage contact and discourage interpenetration.
Hand-object distance is central to grasping. To model this, we learn a Grasping Field [] that characterizes every point in a 3D space by the signed distances to the surface of the hand and the object. The hand, the object, and the contact area are represented by implicit surfaces in a common space. The Grasping Field is parameterized with a deep neural network trained on ObMan's synthetic data.
ObMan's dataset contains hand grasps synthesized by robotics software. However, real human grasps look more varied and natural. Moreover, humans use not only their hands, but also use the body and face during interactions. We therefore capture GRAB [], a dataset of real whole-body human grasps of objects. We use a high-end MoCap system, capture 10 subjects interacting with 51 objects, and reconstruct 3D SMPL-X [
] human meshes interacting with 3D object meshes, including dynamic poses and in-hand manipulation. We use GRAB to train GrabNet, a deep network that generates 3D hand grasps for unseen 3D objects.
Members
Publications